"Nam et ipsa scientia potestas est" (For knowledge itself is power)
Francis Bacon, "Meditationes Sacrae" - 1597
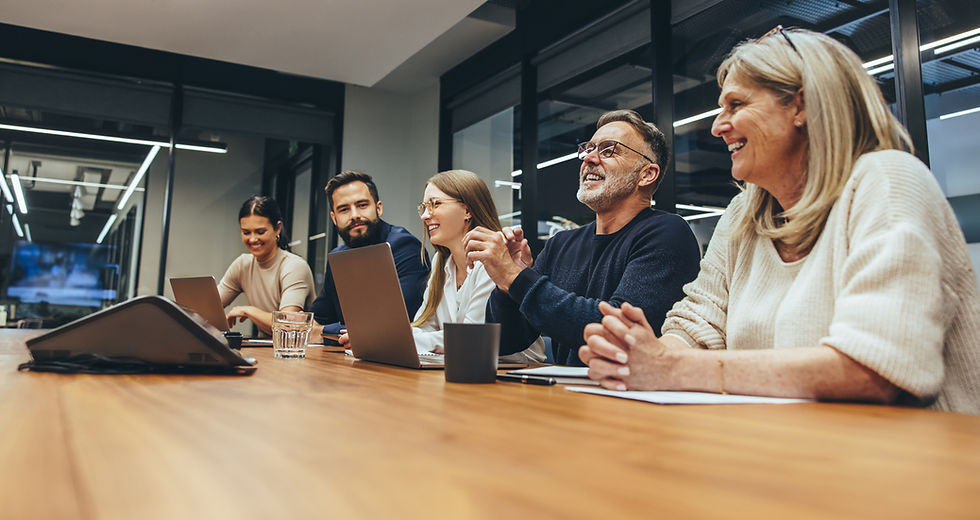
V.3 Updated 21 Jan 2025
Introduction
AI systems, embodying vast amounts of knowledge, wield immense power to transform industries, economies, and our daily lives. However, with this power comes the profound responsibility for us to manage the inherent risks of deploying such transformative and exploratory technologies.
This article explores the expansive landscape of AI risk management, extending beyond the current focus on Large Language Models (LLMs) and Generative AI. By examining the historical evolution of AI risk management, analysing current frameworks and methodologies, and contemplating future challenges, we aim to provide a comprehensive guide for understanding where we have been, where we are now, and where we are going. This insight is crucial for scholars, practitioners, and policymakers to navigate the complexities of AI responsibly.
Context
AI development is not confined to theoretical research; it has real-world implications affecting privacy, security, employment, and ethical norms. Technical developments are outpacing policy and ethical frameworks. New models and papers emerge weekly, making it challenging to discern practical advancements from hyperbole. This article serves as a guide to help individuals and organisations understand AI risk management. As we enter the "Age of AI," incorporating AI risk management into organisational frameworks and boardroom discussions is imperative to ensure accountability and foresight.
The stakes in AI development and deployment are astronomically high. We are not merely optimising processes; we are dealing with systems that could significantly influence the trajectory of human civilisation. There are other papers that talk about the limitations of the architectural design of models and systems - that is not the aim of this paper - AI is here, and AI risk management needs to be taken seriously and understood. Models, use cases, and frameworks will come and go, but AI risk management will need to increase and be ongoing. By fostering a broader understanding of risks, we can work towards developing AI systems that are powerful, efficient, safe, and aligned with human values.
Foundations of AI Risk Management
The foundations of AI risk consideration trace back to the early days of computing and even earlier speculative thought about intelligent machines:
Samuel Butler's "Darwin Among the Machines" (1863): Butler speculated about machines evolving intelligence through natural selection, hinting at potential conflicts between humans and intelligent machines [1]. While not discussing AI as we know it today, his work raised early questions about the relationship between humans and machines.
E.M. Forster's "The Machine Stops" (1909): Forster's story demonstrates remarkable foresight in exploring the potential pitfalls of a society overly reliant on technology [2].
Karel Čapek's "R.U.R." (Rossum's Universal Robots) (1920): Čapek introduced the term "robot" and explored themes of artificial beings revolting against their creators [3]. This play highlighted the potential consequences of creating intelligent machines without considering the risks.
Timeline of AI Risk Management
Early Conceptualizations of AI Risk
Alan Turing's seminal paper, "Computing Machinery and Intelligence" (1950): Turing explored the capabilities of machines to exhibit intelligent behavior indistinguishable from humans [4]. While Turing did not explicitly address AI risks, his work set the stage for future contemplation of the implications of machine intelligence.
In 1965, I.J. Good introduced the concept of an "intelligence explosion" in his paper "Speculations Concerning the First Ultraintelligent Machine" [5]. Good posited that an ultraintelligent machine could design even more capable machines, leading to a rapid, uncontrollable advancement in AI capabilities. This idea raised concerns about the ability to predict and control superintelligent AI systems—a challenge that persists today.
Expanding the Scope in the Late 20th Century
As AI matured as a discipline, discussions expanded to include practical and ethical considerations:
Algorithmic Bias and Fairness: Researchers recognised that AI systems could perpetuate or exacerbate societal biases. If not addressed, these biases could lead to unfair treatment of individuals or groups based on race, gender, or other attributes [6].
Privacy Concerns: The vast data requirements of AI systems heightened privacy issues, prompting discussions about data protection and user consent [7]. Ensuring that AI respects privacy rights became a critical aspect of risk management.
Societal Inequalities: Awareness grew around AI's potential impact on employment and wealth distribution, possibly widening socio-economic gaps.
Eliezer Yudkowsky emerged as a significant figure in AI safety in the late 1990s, formalising the "Friendly AI" concept in the early 2000s [8]. His work emphasised aligning AI objectives with human values, influencing subsequent research on AI alignment.
Formalizing Risk Frameworks in the Early 21st Century
The early 2000s marked a shift towards formal risk analysis and management frameworks:
Nick Bostrom's Contributions: Bostrom's works, including "Ethical Issues in Advanced Artificial Intelligence" [9] and later "Superintelligence: Paths, Dangers, Strategies" (2014) [10], systematically examined potential risks of AI development. He introduced concepts like instrumental convergence and the orthogonality thesis.
Yudkowsky's Advancements: In "Artificial Intelligence as a Positive and Negative Factor in Global Risk," Yudkowsky discussed AI's potential to significantly benefit or harm humanity, underscoring the importance of careful development and initial conditions [11].
The 2010s: Interdisciplinary and Multidimensional Approaches
The 2010s saw the rise of interdisciplinary research institutions:
Establishment of Key Institutes:
Machine Intelligence Research Institute (MIRI): Focused on the technical aspects of AI safety, including decision theory and value alignment [12].
Future of Humanity Institute (FHI): Explored existential risks, integrating philosophy, mathematics, and policy analysis [13].
OpenAI and DeepMind's Safety Team: Both organisations have significantly influenced the AI safety field. OpenAI emphasises responsible development and use of AI [14], while DeepMind's safety research focuses on robustness, verification, and value alignment [15].
Advances in AI Alignment Research:
Stuart Russell's Human-Compatible AI: Advocated for AI systems that understand and act upon human intentions, even when not explicitly specified [16]. This approach seeks to ensure that AI systems remain beneficial as they become more capable.
Risk Taxonomies and Classifications:
Roman V. Yampolskiy's Taxonomy: Provided structured classifications of potential AI risks, facilitating targeted mitigation strategies [17].
Contemporary Frameworks and Methodologies
Recent years have ushered in regulation and sophisticated frameworks for assessing and managing AI risks:
OECD AI Principles (2019): These principles emphasise the importance of transparency, robustness, accountability, and human rights in AI development and were updated in 2024. They have been adopted by over 40+ countries, providing a critical foundation for international AI governance [18].
EU AI Act (2024): The EU AI Act creates a comprehensive regulatory framework for AI within the European Union. It aims to ensure that high-risk systems meet safety and transparency requirements but does not cover AI systems used solely for military, national security, research and non-professional purposes [19].
Transformative AI Risk Analysis (TARA): Critch and Russell's framework offers a comprehensive approach to evaluating societal-scale risks [20]. It considers factors like multi-agent dynamics and uncertainties in decision-making processes.
Sociotechnical Safety Evaluation: Weidinger et al. advocate for holistic evaluations of AI systems, integrating technical safety with socio-ethical considerations such as the potential for misinformation and impacts on human autonomy [21].
MIT AI Risk Repository (2024): A comprehensive database of risks from AI systems; the project reviewed 43 AI frameworks produced by research, industry, and government organizations and identified 777 risks in total. It has been updated in 2025 to include 1,000 risks and 56 existing frameworks [22].
NIST AI Risk Management Framework (RMF): Composed of four functions—govern, map, measure, and manage—NIST's RMF provides a structured approach to enhance AI system reliability, focusing on accountability and risk assessment [23].
To enhance AI system reliability, several technical methods are being employed:
Formal Verification Methods: Techniques to mathematically prove properties about algorithms and systems, ensuring they behave as intended under specified conditions [24].
Adversarial Training: Improving model robustness by exposing them to challenging scenarios during training, helping them perform reliably in the face of unexpected inputs [25].
Robust Optimization Techniques: Designing algorithms that maintain performance under a range of conditions, enhancing their resilience to uncertainties [26].
Current Approaches in AI Risk Management
Modern AI risk management emphasises:
Adaptive Governance Models: Implementing regulatory frameworks that evolve with technological advancements, informed by foresight and scenario planning. Examples include initiatives by OECD and the ongoing development of the EU AI Act [18, 19].
Interdisciplinary Collaboration: Bridging computer science, ethics, sociology, and policy disciplines to address the multifaceted nature of AI risks. Organizations like Partnership on AI have been crucial in fostering this collaboration [27].
Stakeholder Engagement: Ensuring diverse participation in AI governance. Effective communication of technical aspects to non-technical audiences is crucial to build understanding and trust.
Ethical and Value Alignment: Developing methodologies to align AI behaviour with complex human values, acknowledging challenges like differing cultural norms and moral uncertainties [28].
Future Directions and Challenges
Looking ahead, key challenges include:
Scalable AI Alignment: Developing solutions to align advanced AI systems with human values as they become more capable [29]. This includes ongoing research into methods that ensure AI systems act in ways that are beneficial to humanity.
Robustness and Verification: Enhancing the reliability of AI systems through advanced verification methods, adversarial training, and robust optimization [30].
Global Coordination Mechanisms: Establishing international agreements and organisations to manage AI deployment, preventing arms races or unilateral actions that could exacerbate risks. UNESCO’s AI Ethics Recommendation (2021) is an example of such an effort [31].
Ethical Frameworks for Autonomous Systems: Refining ethical guidelines for AI decision-making, integrating cross-cultural perspectives to respect diverse values [32].
Author's Note:
AI should give people more freedom, not less. It must be designed to operate transparently so individuals can understand how it works and how it will affect them. This understanding will enable them to make decisions (preferably evidence-based) about whether and how to use it. Our efforts in AI risk management are vital as we navigate this transformative era. Embedding robust AI Risk frameworks and tools into organisations are critical steps, and their importance will only grow.
The actions we take today will significantly influence AI's impact on humanity's future. We recommend organisations implement a sociotechnical AI framework because AI systems are not merely sets of algorithms; they exist within and impact human, social, and organisational systems. Ignoring the social dimensions will lead to incomplete risk assessments, potentially resulting in serious unintended consequences, including ethical breaches, user distrust, and societal harm. Including a sociotechnical perspective helps ensure that AI systems are not only technologically sound but also socially responsible and contextually appropriate. This holistic view is crucial for building AI that serves society in a trustworthy and equitable manner.
References
Butler, S. (1863). "Darwin Among the Machines." The Press, Christchurch, New Zealand.
Forster, E. M. (1909). "The Machine Stops." Oxford and Cambridge Review.
Čapek, K. (1920). R.U.R. (Rossum's Universal Robots).
Turing, A. M. (1950). "Computing Machinery and Intelligence." Mind, 59(236), 433-460.
Good, I. J. (1965). "Speculations Concerning the First Ultraintelligent Machine." Advances in Computers, 6, 31-88.
Friedman, B., & Nissenbaum, H. (1996). "Bias in Computer Systems." ACM Transactions on Information Systems, 14(3), 330-347.
Sweeney, L. (1997). "Weaving Technology and Policy Together to Maintain Confidentiality." Journal of Law, Medicine & Ethics, 25(2-3), 98-110.
Yudkowsky, E. (2001). Creating Friendly AI. Singularity Institute for Artificial Intelligence.
Bostrom, N. (2003). "Ethical Issues in Advanced Artificial Intelligence." In Cognitive, Emotive and Ethical Aspects of Decision Making in Humans and in Artificial Intelligence (Vol. 2, pp. 12-17).
Bostrom, N. (2014). Superintelligence: Paths, Dangers, Strategies. Oxford University Press.
Yudkowsky, E. (2008). "Artificial Intelligence as a Positive and Negative Factor in Global Risk." In Bostrom, N., & Ćirković, M. M. (Eds.), Global Catastrophic Risks (pp. 308-345). Oxford University Press.
Machine Intelligence Research Institute (MIRI).
Future of Humanity Institute (FHI).
OpenAI. (2015). About OpenAI.
DeepMind Safety Research.
Russell, S. (2019). Human Compatible: Artificial Intelligence and the Problem of Control. Viking.
Yampolskiy, R. V. (2016). "Taxonomy of Pathways to Dangerous Artificial Intelligence." In Workshops at the Thirtieth AAAI Conference on Artificial Intelligence.
OECD. (2019). OECD AI Principles.
European Commission. (2024). Artificial Intelligence (AI Act). Retrieved from
Critch, A., & Russell, S. (2023). "The Transformative AI Risk Analysis." Journal of Artificial Intelligence Research, 70, 1001-1050.
Weidinger, L., et al. (2023). "Sociotechnical Safety Evaluation of AI Systems." AI and Society, 38(1), 123-145.
Slattery, P., Saeri, A. K., Grundy, E. A. C., Graham, J., Noetel, M., Uuk, R., Dao, J., Pour, S., Casper, S., & Thompson, N. (2024). "MIT, A Systematic Evidence Review and Common Frame of Reference for the Risks from Artificial Intelligence, 2024."
National Institute of Standards and Technology (NIST). (2023). Artificial Intelligence Risk Management Framework (AI RMF).
Amodei, D., et al. (2016). "Concrete Problems in AI Safety." arXiv preprint arXiv:1606.06565.
Goodfellow, I. J., Shlens, J., & Szegedy, C. (2015). "Explaining and Harnessing Adversarial Examples." In International Conference on Learning Representations.
Ben-Tal, A., El Ghaoui, L., & Nemirovski, A. (2009). Robust Optimization. Princeton University Press.
Partnership on AI. (2016).
Gabriel, I. (2020). "Artificial Intelligence, Values, and Alignment." Mind, 129(516), 1069-1094.
Hadfield-Menell, D., et al. (2016). "Cooperative Inverse Reinforcement Learning." In Advances in Neural Information Processing Systems (pp. 3909-3917).
Amodei, D., et al. (2016). "Concrete Problems in AI Safety." arXiv preprint arXiv:1606.06565.
UNESCO. (2021). "Recommendation on the Ethics of Artificial Intelligence." Retrieved from
Awad, E., et al. (2018). "The Moral Machine Experiment." Nature, 563(7729), 59-64.