AI and Certainty
- Christopher Foster-McBride
- Jul 26, 2024
- 6 min read
Updated: Jan 21
As AI, particularly multimodal frontier models like GPT-4o, Gemini 2.0, or Claude’s 3.5 Sonnet, continue to reshape industries, and awareness of agents and multi-agent systems grows, executives face crucial decisions about how these technologies are developed and implemented. However, a fundamental question often remains unaddressed for boards: When we invest in AI solution X, can we guarantee the X output/benefit?
In this article, we discuss why this question is paradoxical and explore the technical and philosophical roots of AI uncertainty, and outline ten examples of how executives can have a more sophisticated approach to AI deployment and derisk engagements for their organisations.
Absolute certainty remains an ideal rather than an achievable goal - all knowledge is ultimately provisional. We conclude that while absolute certainty remains elusive, executives can harness the power of AI responsibly to guide their decisions by:
Understanding the probabilistic nature of LLMs /MMFMs
Implementing robust oversight and verification processes, and constantly reflecting on risk appetite as a leader and from an organisation standpoint
Fostering a culture of transparency and continuous improvement
Context
LLMs operate on probabilistic principles, utilising vast datasets to generate responses. During text generation, these models employ sampling methods (like Temperature, Top-P, or Top-K) to produce diverse and creative outputs. This probabilistic nature means that while LLMs can provide highly accurate and useful information, they cannot offer absolute certainty in their responses.
The uncertainty inherent in these AI systems raises significant ethical questions, particularly in high-stakes scenarios such as healthcare diagnostics or financial decision-making, where hallucinations (models confabulating) can have serious implications. To be useful, executives must consider model selection, and techniques to ground AI in contextual knowledge and have a deep understanding of the well-documented and evidence-based AI risks. This necessitates robust AI Risk Assessment frameworks and clear communication about AI limitations to all stakeholders.
As part of our strategy, Digital Human Assistants advocate for Human-in-the-loop (HITL) approaches in many of our AI builds. In our agentic work, we see AI agents and humans working alongside each other to iteratively complete tasks. We see AI as a helpful assistant that shapes content, guided by humans rather than replacing them.
Given that we still have some way to go to reach the tipping point of consistent output from top-performing AI models (see latest benchmarks here to get a sense of how they perform in key areas), this iterative sense-checking in workflows allows oversight and ensures that humans can correct content promptly and take ownership of the output.
Absolute Certainty: Let's Get Philosophical
‘I want you to build this AI solution for me… and I want you to guarantee that this works 100%.’
Sound familiar? It’s every AI professional's worst nightmare.
But why? The answer isn’t rooted in Data Science but in Philosophy and is an all-too-human issue.
As an executive, if you want to engage with concepts like ‘absolute certainty,’ there are a few things you need to know… and doing so will give you comfort in dealing with, well, everything where we rely on knowledge and justification (including areas beyond AI).
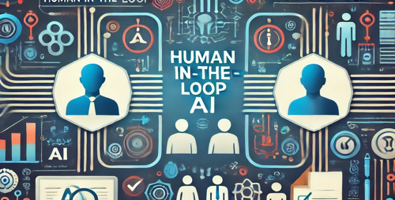
A quick Philosophy lesson: Absolute Certainty
Going back to the ancient sceptic philosophers, humanity has wrestled with developing systematic challenges to the possibility of certain knowledge (e.g., Agrippa's Trilemma, which predates the Münchhausen Trilemma by nearly two millennia is an important concept in epistemology and wroth researching).
Hans Albert (1921-2023), a German philosopher, explicitly formulated the Münchhausen Trilemma, building on the Agrippa Trilemma. He highlighted the impossibility of achieving absolute justification for any knowledge claim, leading to the conclusion that all knowledge is ultimately provisional (this includes the knowledge in the information and data used for pre-training LLMs and therefore the output we often assume to be knowledge produced by AI models).
Examples of the Münchhausen Trilemma
When we attempt to prove any truth, we are faced with three options, all of which are problematic:
Infinite Regress: This is where each proof requires a further proof, ad infinitum.
Circular Reasoning: This is where the proof relies on statements that have not been proven themselves, creating a circular argument.
Axiomatic Argument (or Foundationalism): This is where the proof relies on accepted axioms or "self-evident" truths.
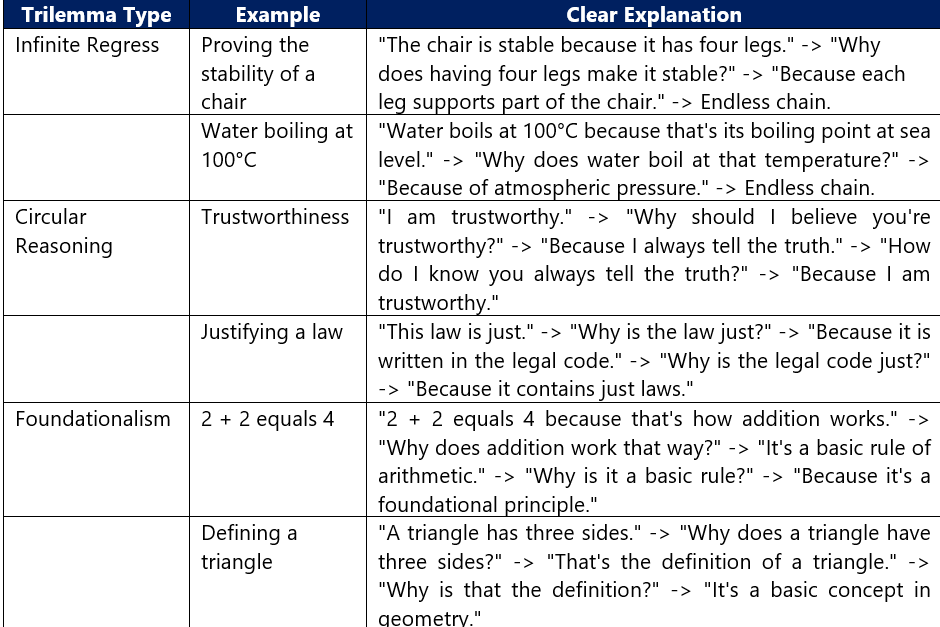
How does this relate to AI and executives?
While many great thinkers (see notes below) have tried to wrestle and unpack this, absolute certainty remains an ideal rather than an achievable goal and recent strategies aim to build more reliable and justified knowledge.
Why flag this? Why care? For executives, this knowledge fosters a more sophisticated approach to AI deployment. It encourages a balance between leveraging AI capabilities and maintaining a critical, thoughtful approach to its limitations and ethical implications. This nuanced understanding can lead to more responsible, effective, and sustainable AI integration in business operations.
Ten areas where this applies to board level thinking:
Decision-Making Under Uncertainty:
AI systems often make decisions based on data and algorithms. The trilemma highlights that perfect certainty is unattainable.
Executives need to be comfortable with probabilistic reasoning and decision-making under uncertainty.
Ethical Considerations:
When AI makes high-stakes decisions, understanding the limits of justification helps in setting appropriate confidence levels and safeguards.
It encourages a more nuanced approach to AI ethics and governance.
Transparency and Explainability:
The trilemma underscores the importance of being able to explain AI decisions, even if they can't be proved with absolute certainty.
This aligns with growing demands for explainable AI (XAI) in regulated industries.
Risk Management:
Recognising the limitations of knowledge claims helps in better risk assessment and mitigation strategies for AI deployments.
It encourages robust testing and validation processes.
Avoiding Overconfidence:
Understanding the trilemma can prevent overreliance on AI outputs, encouraging human oversight and intervention when necessary.
Continuous Learning and Adaptation:
The infinite regress aspect of the trilemma aligns with the need for continuous learning and updating of AI models.
Stakeholder Communication:
Executives can use this knowledge to better communicate the capabilities and limitations of AI systems to stakeholders, setting realistic expectations.
Legal and Regulatory Compliance:
In sectors with strict regulations, understanding the limits of justification helps in creating appropriate documentation and audit trails for AI decisions.
Innovation and Research Direction:
It can guide research and development efforts towards more robust and adaptable AI systems that acknowledge uncertainty.
Strategic Planning:
Long-term AI strategies can benefit from this philosophical insight, promoting flexibility and adaptability in technological roadmaps.
Author: Christopher Foster-McBride, CEO, Digital Human Assistants
Our mission is to use AI and IA to support those that support others. Book an appointment for a conversation here today.
Notes:
Large Language Models (LLMs), such as GPT-4o, are advanced AI systems trained on vast amounts of text data, capable of generating human-like text, answering questions, and performing various language-related tasks.
Notes on the Philosophical History of the Agrippa and Münchhausen Trilemmas
Sextus Empiricus (c. 160 – c. 210 AD): As an ancient Greek philosopher and physician, Sextus Empiricus is one of the most significant sources of ancient scepticism. He articulated the five tropes of Agrippa, including the trilemma, which challenges the possibility of certain knowledge. His works have influenced the development of scepticism in Western philosophy, emphasising the need for suspension of judgment (epoché) when faced with the trilemma.
René Descartes (1596-1650): Descartes is often considered the father of modern philosophy. He attempted to overcome scepticism by finding an indubitable foundation for knowledge, famously stating, "Cogito, ergo sum" ("I think, therefore I am"). Descartes acknowledged the challenge of infinite regress but sought to establish certain knowledge through rationalism and the existence of a benevolent God who would not deceive us about the nature of reality.
Immanuel Kant (1724-1804): Kant sought to address the limitations of human knowledge by distinguishing between phenomena (things as they appear) and noumena (things in themselves). He argued that while we cannot have direct knowledge of the noumenal world, we can have reliable knowledge of the phenomenal world through the categories of understanding. Kant's transcendental idealism provided a framework for understanding the limits of human knowledge and offered a way to navigate the trilemma by focusing on the conditions that make knowledge possible.
Ludwig Wittgenstein (1889-1951): Wittgenstein's later philosophy, particularly in "On Certainty," addresses the foundations of knowledge. He argued that certain basic beliefs form the groundwork of our language games and practices, and these beliefs are not subject to doubt in the same way as empirical claims. Wittgenstein's approach suggests that the trilemma can be mitigated by recognising that some beliefs are foundational to our language and action, and these do not require further justification.
Hans Albert (1921-2023): Hans Albert, a German philosopher, explicitly formulated the Münchhausen Trilemma, building on the Agrippa Trilemma. He highlighted the impossibility of achieving absolute justification for any knowledge claim, leading to the conclusion that all knowledge is ultimately provisional. Albert's work has reinforced the importance of critical rationalism, where knowledge claims are always open to revision and must be subjected to continuous scrutiny and testing.
Who was Karl Friedrich Freiherr von Münchhausen (1720-1797)? Münchhausen gained fame for his captivating stories, which he claimed to have “collected through his travels.” One of the most renowned stories involves a horseback riding escapade gone awry. As the narrative goes, he found himself stuck in a swamp during this adventure. Münchhausen’s audacious claim that he rescued both himself and his horse from the predicament by pulling his own hair has fuelled scepticism and left listeners intrigued and doubtful about the baron’s storytelling prowess.